Workshop "Statistical methods for safety and decommissioning"
- When & where
- Presentation of the workshop
- Confirmed speakers and slides
- Call for posters
- Registration
- Agenda
- Abstracts
When & where
The workshop will take place on November 21-22, 2022 at Campus Hannah Arendt, Université d'Avignon, Avignon (Bâtiment Nord, Amphithéâtre 2 E 07, 74 rue Louis Pasteur, 84000 Avignon). It is located on the centre of Avignon, close to the railway station (1km).
Presentation of the workshop
This conference aims to bring together researchers and engineers from the academic and industrial sectors to address the use of statistical methods for industrial safety and decommissioning challenges. Four topics will be covered: spatial statistics for pollution characterization, measurements and uncertainty, metamodelling techniques and risk analysis techniques.
A poster and drinks session will be held at the end of the first day to allow participants to present their work.
The languages will be French and English.
Organizers: Marielle Crozet (CEA Marcoule, CETAMA), Céline Helbert (Ecole Centrale de Lyon, Institut Camille Jordan), Bertrand Iooss (EDF R&D), Céline Lacaux (Université d'Avignon, Lab. de Mathématiques d'Avignon).
Sponsored by: CEA, CETAMA, LMA, Avignon Université, GDR MASCOT-NUM
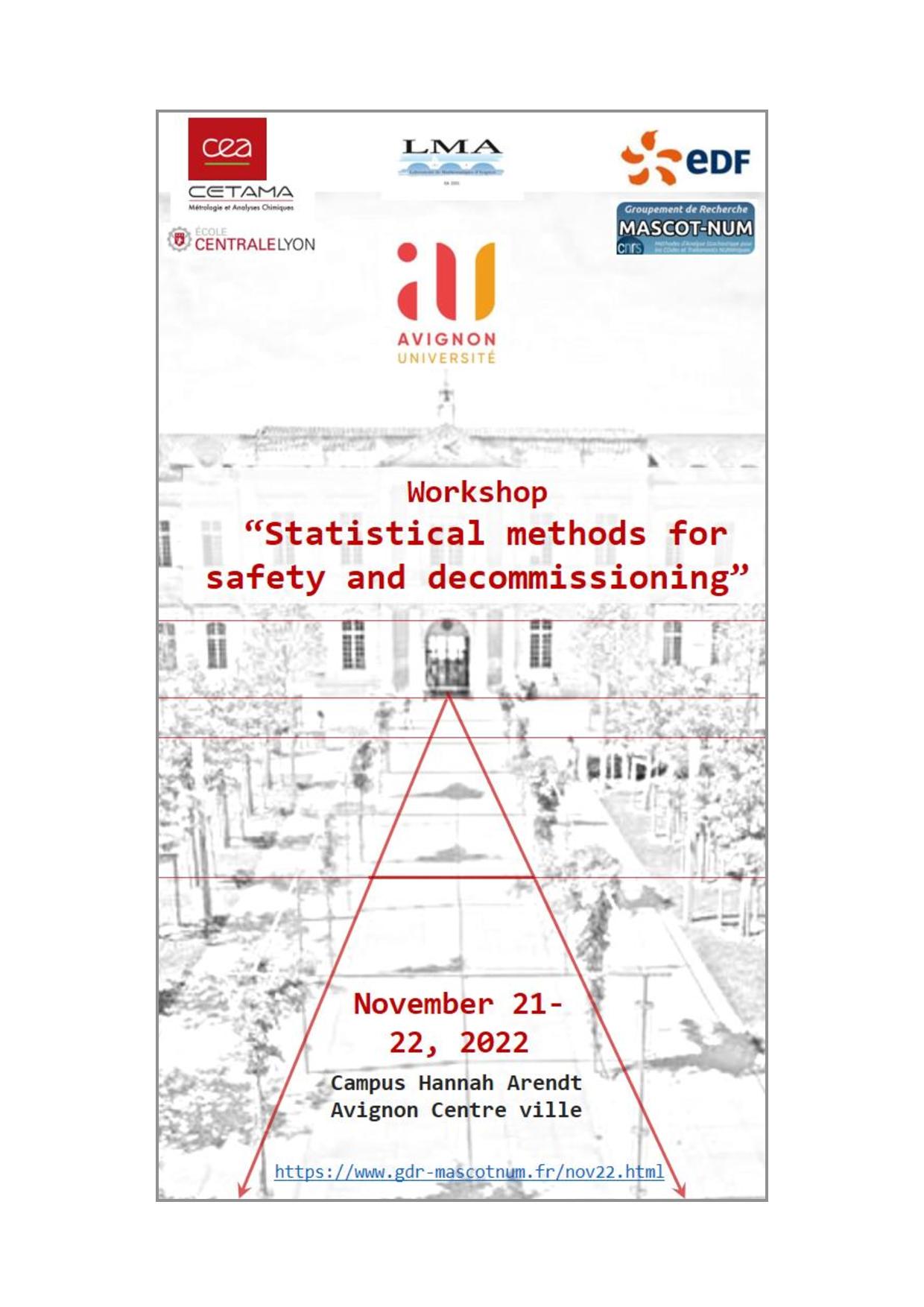
Confirmed speakers and slides
- Sophie Ancelet (IRSN Fontenay-aux-Roses): Hierarchical modeling and Bayesian statistics for a better consideration of uncertainties when estimating radiation-related risks - slides
- François Bachoc (Institut de Mathématiques de Toulouse): Introduction to Gaussian process with inequality constraints - Application to coast flooding risk - slides
- Emanuele Borgonovo (Bocconi University): Reliability importance measures: From local to global - slides
- Nicolas Bousquet (EDF R&D): Risk, uncertainty, and robust decision-making: an attempted Introduction - slides
- Aloïs Clément (CEA Valduc): Bayesian Approach for Multigamma Radionuclide Quantification Applied on Weakly Attenuating Nuclear Waste Drums - slides
- Jean-Philippe Dancausse, Magali Saluden et Catherine Eysseric (CEA Marcoule, DES/DDSD): Expected contributions of statistical methods to nuclear decommissioning of CEA facilities - slides
- Michele Désenfant (LNE): A measurement process is not a deterministic algorithm! - slides
- Yvon Desnoyers (Geovariances): Smart use of the variogram to explore spatial data, to break down variance contributions and to model radiological contaminations - slides
- Mélanie Ducoffe (Airbus Group): Verification of overestimation and partial monotonicity for neural network-based surrogate for aircraft braking distance estimation - slides
- Mitra Fouladirad (Ecole Centrale de Marseille): Wind speed modelling with stochastic processes for wind turbine production study - slides
- Amandine Marrel (CEA Cadarache, DES/DER): Statistical approach in nuclear safety problems: recent advanced around sensitivity analysis and metamodeling - slides
- Claude Norman, Sarah Michalak (IAEA) and Tom Burr (Los Alamos Nat. Lab.): Reconcilier l’estimation d’incertitudes de mesure ascendante basée sur le GUM (Guide for the Expression of Uncertainty in Measurement) et l’estimation descendante basée sur le modèle statistique de l’IAEA - slides
- Thomas Romary (Mines ParisTech): Scenario reduction for uncertainty quantification in Uranium in situ recovery - slides
Call for posters
A poster session is organised on November, 21. If you want to participate please send an email to Céline Lacaux with the poster title, the authors and a few words on the subject.
- Jean Baccou (IRSN): Modèle probabiliste de prédiction de chemins de fissuration : application aux matériaux cimentaires - mascot-nov22_baccou-poster.pdf
- Vincent Chabridon (EDF R&D): Robustness assessment of reliability-oriented quantities of interest for the safety of industrial structures using the info-gap framework - mascot-nov22_chabridon-poster.pdf
- Chantal de Fouquet (Ecole des Mines de Paris): Estimation du panache de contamination de la T22 à proximité de Tchernobyl. Comparaison de méthodes - mascot-nov22_defouquet-poster.pdf
- Clément Gauchy (CEA Saclay, DES/DM2S): Uncertainty quantification and global sensitivity analysis of seismic fragility curves using kriging
- Raphaël Perillat (Phimeca): Réduction de dimension et méta-modélisation pour la prise en compte des incertitudes en crise nucléaire - mascot-nov22_perillat-poster.pdf
- Martin Wieskotten (CEA Marcoule et Univ. Avignon): Traitement géostatistique des résultats de mesure pour la caractérisation radiologique dans le cadre de l’assainissement/démantèlement de sites nucléaires - mascot-nov22_wieskotten-poster.pdf
Registration
Registration is closed
Agenda
Abstracts
- Jean Baccou, K. Pele, F. Perales, L. Daridon, J. Liandrat, T. Le Gouic, Y. Monerie - Modèle probabiliste de prédiction de chemins de fissuration : application aux matériaux cimentaires - Le prolongement de la durée d’exploitation des centrales nucléaires françaises soulève de nombreuses questions de recherche sur le vieillissement des ouvrages de génie civil nucléaire. Les phénomènes intervenant lors de ce vieillissement sont principalement liés au développement de pathologies, notamment les réactions de gonflements internes. Ces phénomènes sont susceptibles en particulier de dégrader le béton et de conduire à l’apparition de fissures dans les structures touchées. Afin d’étudier les différentes pathologies et leurs conséquences sur la structure, des simulations numériques à l’aide de codes mécaniques à champ complet sont réalisées. Cependant, chaque simulation est coûteuse en temps calcul, ce qui est un frein dans les applications industrielles nécessitant un grand nombre de simulations. Pour limiter les coûts, on s’intéresse dans ce travail à la construction d’un modèle rapide à évaluer pour la prédiction de chemins de fissuration. Le nouveau modèle est appliqué sur une expérience classique dans le domaine des matériaux cimentaires, une poutre en flexion trois points.
- François Bachoc: Introduction to Gaussian process with inequality constraints, Application to coast flooding risk - In Gaussian process modeling, inequality constraints enable to take expert knowledge into account and thus to improve prediction and uncertainty quantification. Typical examples are when a black-box function is bounded or monotonic with respect to some of its input variables. We will show how inequality constraints impact the Gaussian process model, the computation of its posterior distribution and the estimation of its covariance parameters. An example will be presented, where a numerical flooding model is monotonic with respect to two input variables called tide and surge.
- Nicolas Bousquet: Risk, uncertainty, and robust decision-making: an attempted Introduction - This introductory presentation will attempt to provide an overarching framework for many of the different contributions that will be presented. Reminders on the formalization of risk and decision, the evidence of the crucial influence of uncertainties and the need to study robustness will be illustrated by case studies from different engineering domains. Approaches using penalized models and non-asymptotic statistical tools will also be presented to partially solve these problems. I will conclude with some proposals for challenges to improve the treatment of uncertainties and decision making, in which many contributions seem to be a priori included.
- Emanuele Borgonovo: Reliability importance via optimal transport - In this presentation, we will review the notion of reliability importance. We will discuss both local and global approaches. We will then focus on the application of the theory of optimal transport for the construction of reliability importance measures, with applications in safety and risk assessment
- Vincent Chabridon, Antoine Ajenjo, Emmanuel Ardillon, Scott Cogan, Emeline Sadoulet-Reboul: Robustness assessment of reliability-oriented quantities of interest for the safety of industrial structures using the info-gap framework - Structural reliability is of particular interest for risk-sensitive industrial applications such as power generation where system performance, and therefore safety, is subject to uncertainty. In this context, safety is assessed by estimating reliability-oriented quantities of interest such as a low probability of failure or a high-order quantile on a specific output variable of interest. High-risk system models are typical cases where epistemic uncertainty can be found as they often represent events that are rarely or never encountered. However, the potential impact of lack of knowledge must still be accounted for in order to make an informed decision on the safety of the system. For this purpose, the info-gap methodology is applied to reliability quantities of interest to provide a robustness metric defined as the amount of uncertainty that a nominal model may tolerate without changing a design decision. This framework implies a large number of evaluations of the reliability quantity of interest. Therefore, its implementation requires efficient strategies for reducing the huge computational burden induced both by the info-gap formulation and the reliability problem.
- Aloïs Clément: Bayesian Approach for Multigamma Radionuclide Quantification Applied on Weakly Attenuating Nuclear Waste Drums - See https://ieeexplore.ieee.org/document/9500209
- Chantal de Fouquet, Le Coz M., Freulon X., Pannecoucke L.: Estimation du panache de contamination de la T22 à proximité de Tchernobyl. Comparaison de méthodes - A l'aval hydraulique de l’une des tranchées d'enfouissement des matériaux contaminés à proximité de la centrale de Tchernobyl, les activités en 90Sr dans les eaux souterraines sont fortement contrastées dans l’espace et dans le temps. Pour caractériser la non-stationnarité spatio-temporelle des activités, des séries de simulations d'écoulement et de transport sont effectuées en randomisant les paramètres d’entrée d’un modèle à base physique ; puis des modèles empiriques non stationnaires de variogramme ("variogramme numérique", cf. Pannecoucke, 2020 ; Pannecoucke et al., 2020) sont calculés sur les sorties de ces simulations. Plusieurs méthodes d'estimation géostatistique ou leurs approximations sont ensuite mises en œuvre (krigeage avec variogramme linéaire proportionnel, variogramme numérique ou espérance conditionnelle en modèle gaussien anamorphosé) et la précision de l'estimation ainsi que la cohérence des résultats sont évaluées.
- Michele Desenfant : A measurement process is not a deterministic algorithm! - We are sensitive to the temporal, spatial variation, the variation of the models but let's not forget the uncertainty associated with a measurement result.
- Yvon Desnoyers: Smart use of the variogram to explore spatial data, to break down variance contributions and to model radiological contaminations - Before performing any modelling and estimation calculation, the first and main part of any geostatistical study is to intensively work on the dataset, to explore and validate it. In addition to classical statistics tools such as basemap, histogram and scatter plot, the variogram strengthens this analysis by the identification of spatial inconsistencies, by the decomposition of the different variability contributions (between sample duplicates, measurement replicates and spatial variability) and consequently by the interpretation and modelling of the spatial structure. Illustrated on several radiological contamination cases, this presentation will describe and detail the advanced and smart use of the variogram.
- Mélanie Ducoffe: Verification of overestimation and partial monotonicity for neural network-based surrogate for aircraft braking distance estimation - In recent years, we have seen the emergence of safety-related properties for regression tasks in many industries. For example, numerical models have been developed to approximate the physical phenomena inherent in their systems. Since these models are based on physical equations, the relevance of which is affirmed by scientific experts, their qualifications is carried out without any problems. However, as their computational costs and execution time prevent us from embedding them, the use of these numerical models in the aeronautical domain remains mainly limited to the development and design phase of the aircraft. Thanks to the current success of deep neural networks, previous works have already studied neural network-based surrogates for the approximation of numerical models. Nevertheless, these surrogates have additional safety properties that need to be demonstrated to certification authorities. In this talk, we will examine two specifications that arise for a neural network used for aircraft braking distance estimation: over-prediction of the simulation model and partial monotonicity. We will explore two ongoing research directions to address them: probabilistic evaluation using Bernstein-type deviation inequalities and formal verification of neural networks using mixed integer programming and linear relaxation based perturbation analysis.
- Mitra Fouladirad: Wind speed modelling with stochastic processes for wind turbine production study - The main issue in wind turbine production, reliability and degradation analysis is the wind speed short and long-term forecasting for different geographical sites. Indeed, according to different positions of wind turbines in a wind farm they are not subject to the same wind speed and the production or reliability analysis based on an average wind speed value is not very sensible. Although, geographical wind data are available, for an efficient production or reliability analysis, it is essential to model the wind speed and to be able to generate data faster than CFD methods. In this study, based on prior information and clustering methods wind data are analysed and an Ornstein-Uhlenbeck process with covariates is proposed for wind modelling. The validation is analysed through depth functions and bootstrap methods.
- Clément Gauchy: Uncertainty quantification and global sensitivity analysis of seismic fragility curves using kriging - Recent advances in numerical simulation have allowed the emergence of methodologies for uncertainty quantification of computer codes. This approach is now used for seismic probabilistic safety studies. In this field, the quantity of interest is the probability of failure conditional on the seismic intensity of the mechanical structure. Nevertheless, numerical codes for the simulation of mechanical structures subjected to seismic excitation are generally very expensive in terms of computation time. It is therefore essential to have a statistical learning algorithm that is as economical as possible in terms of the number of evaluations of the appropriate computer code. We propose to use a statistical metamodel using Gaussian process to be able to quickly evaluate the fragility curves and to propagate the uncertainties on the mechanical parameters of the structure on them. After this first step, a sensitivity analysis is performed to study how the uncertainty on the seismic fragility curve of the structure can be divided and allocated to the uncertainties of the mechanical parameters of the structure.
- Amandine Marrel: Statistical approaches in nuclear safety problems: recent advanced around sensitivity analysis and metamodeling - In safety or conception studies on current or future nuclear reactors, we make extensive use of simulation to model, understand and predict the phenomena involved. However, these simulators can take a large number of uncertain input parameters, characterizing the studied phenomenon or related to its physical and numerical modelling. Statistical methods based on Monte Carlo approaches thus provide a rigorous framework to deal with these uncertainties. However, several technical locks have to be addressed, such as the large dimension of the problem (several tens of inputs, e.g.), the complex inputs/output(s) relationship and the high CPU computation time of considered simulators. Sensitivity analysis and metamodeling techniques play a key role to overcome these limitations. The presentation will focus on recent and advanced developments on these topics, illustrated by their application on nuclear use cases.
- Claude Norman and Sarah Michalak: Reconcilier l’estimation d’incertitudes de mesure ascendante basée sur le GUM (Guide for the Expression of Uncertainty in Measurement) et l’estimation descendante basée sur le modèle statistique de l’AIEA - Les Valeurs Cibles Internationales (VCI), plus communément désignées en anglais par l’appellation International Target Values (ITV), sont les valeurs de précision de mesure qui devraient être réalisables dans des conditions industrielles normales pour différentes méthodes de mesure et différents types de matières nucléaires. Ces dernières sont régulièrement revues et publiées par le Département des garanties de l’Agence internationale de l’énergie atomique (AIEA) afin de servir de référence aux exploitants d’installations du cycle du combustible nucléaire. Ces valeurs cibles sont également utilisées comme référence par les inspecteurs des garanties de l’AIEA qui utilisent des méthodes de mesure destructives et non-destructives pour vérifier les déclarations des États. À l’occasion de la réunion des experts internationaux chargés de la préparation de l’édition 2010 des Valeurs Cibles Internationales (ITV-2010), une discussion animée a eu lieu entre les représentants des laboratoires et les évaluateurs de l’AIEA. Alors que les premiers utilisent les méthodes bottom-up (processus ascendant) et la terminologie du Guide pour l’expression de l’incertitude de mesure (GUM), l’AIEA produit les VCI sur la base d’un modèle statistique top-down (processus descendant) exprimant séparément les variances des erreurs aléatoires et systématiques. Afin de réconcilier ces deux approches, un effort de communication important a été entrepris entre les différentes communautés intéressées (laboratoires, inspecteurs et évaluateurs de l’AIEA, Euratom et ABACC, représentants des États pour les garanties…). Cela s’est concrétisé par la rédaction d’un article collaboratif ayant pour titre « Statistical error model-based and GUM based analysis of measurement uncertainties in nuclear safeguards – a reconciliation », habituellement désigné sous le nom de « Reconciliation Paper ». Les conclusions principales de cet article sont d’une part que le GUM et le modèle statistique de l’AIEA ne sont pas contradictoires mais complémentaires et mathématiquement compatibles, et d’autre part que leur synergie peut servir à alimenter les modèles utilisés par chacune des deux approches.
- Raphaël Perillat: Réduction de dimension et méta-modélisation pour la prise en compte des incertitudes en crise nucléaire
- Thomas Romary: Scenario reduction for uncertainty quantification in Uranium in situ recovery - Uranium In Situ Recovery (ISR) is based on the direct leaching of the uranium ore in the deposit by a mining solution. Fluid flow and geochemical reactions in the reservoir are difficult to predict due to geological, petrophysical and geochemical uncertainties. The reactive transport code used to simulate ISR is very sensitive to the spatial distribution of physical and chemical properties of the deposit. Stochastic geostatistical models are used to represent the uncertainty on the spatial distribution of geological properties. The direct propagation of geological uncertainties by multiple ISR mining simulations is intractable in an industrial context, the CPU time needed to perform one ISR numerical simulation being too heavy. We will present a way to propagate geological uncertainties into uranium production uncertainties at a reduced computational cost, thanks to a scenario reduction method.
- Martin Wieskotten: Gostatistical treatment of measurements results for radiological characterisation in the decommissioning of nuclear installation - The decommissioning of nuclear installation offers many challenges in regards to contamination estimation. Due to low levels of contamination, decommissioning datasets often present censored values, where part of the measurement results are only partially known. Geostatistics, as one of the many tools of used for spatial prediction of radionuclides contamination, does not offer a classical methodology for the treatment of these kind of datasets, besides data substitution (consisting in replacing a censored measurement result with an arbitrary value). To answer this problematic, we present here a variation of the data augmentation algorithm, a Bayesian approach allowing treatment of censored data through imputation. This new algorithm takes into account unknown measurement uncertainty through a MCMC approach. To verify its effectiveness we compare it to substitution methods on a real dataset stemming from the decommissioning of the G3 reactor in CEA Marcoule.